A Comprehensive Overview of Data Orchestration in 2024
Written on
Chapter 1: Introduction to Data Orchestration
The realm of data in contemporary organizations is rapidly growing. With an abundance of data comes the challenge of governance, synchronization, and processing. To truly utilize the information being gathered, businesses must dismantle the barriers between data sources and storage systems. Merely adding tools will not resolve these issues—often, it can exacerbate them. To effectively eliminate data silos and manage system overload, organizations require enhanced data governance coupled with data orchestration.
Data orchestration enables businesses to automate and streamline their data processes, transforming valuable information into actionable insights for real-time decision-making. Estimates suggest that a significant portion of data analysis—up to 80%—is dedicated to data ingestion and preparation, indicating that effective data orchestration can significantly reduce processing and scheduling times.
Video Description: A Beginner's Guide to Data Orchestration.
Section 1.1: What is Data Orchestration?
Data orchestration varies at different stages of the data pipeline, but generally, it can be defined as the use of software to eliminate data silos between sources and storage locations, enhancing data accessibility through automation. This process involves improving data collection, preparation, transformation, unification, and delivery.
By investing in the necessary software for data orchestration, companies can better connect their systems and gain access to real-time information.
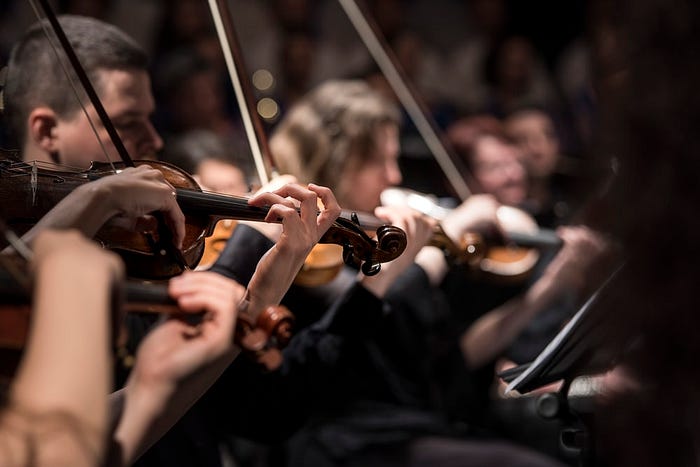
Section 1.2: The Significance of Data Orchestration
As highlighted in the definition, data orchestration encompasses four key processes:
- Data Collection: Comprehensive data orchestration services facilitate data ingestion from critical customer interaction points, often utilizing SDKs and APIs integrated directly with applications.
- Data Preparation and Transformation: After data collection, orchestration services standardize and validate data properties and values, ensuring they conform to a defined schema.
- Data Unification: Orchestration services aid organizations in merging disparate data streams into a cohesive pool, enhancing its overall value. This allows for the creation of unified customer profiles by integrating data from various sources, providing insights into user behavior.
- Delivery and Activation: Unified customer profiles can then be transmitted to the tools utilized daily by teams, such as business intelligence platforms and analytics tools.
Imagine your data pipeline resembling a river, with various tributaries (data sources) feeding into it. Your goal is to construct a reservoir (data warehouse) that generates sufficient energy (insights) to power a nearby town (customers).
Without orchestration, the construction team must manually manage each source, which is time-consuming and labor-intensive. However, with data orchestration, operations can be automated, allowing the team to concentrate on monitoring energy generation and distribution.
Chapter 2: Evolution of Data Orchestration Tools
Video Description: Understanding the Challenges Addressed by Data Orchestration.
Data orchestration tools have evolved to meet the changing needs of organizations. The first generation of tools, such as Airflow, primarily enhanced usability for data scientists by introducing Python support, making it easier to manage pipeline flows.
Second-generation tools, including Dagster and Prefect, focus on data-driven capabilities, detecting data types within Directed Acyclic Graphs (DAGs) and enhancing data awareness. These capabilities can be categorized as:
- Active Approaches: These methods facilitate data transfer between steps and systems.
- Passive Approaches: These wait for external events to trigger tasks, particularly beneficial for continuous model training.
While active orchestration is effective for most applications, passive methods can be useful as data flows become increasingly complex.
Section 2.1: Orchestrated vs. Unorchestrated Data
The distinction between orchestrated and unorchestrated data lies in the ability to operationalize data for future decision-making versus struggling with past data for troubleshooting. Many organizations still grapple with unorchestrated data, with over 87% of businesses exhibiting low business intelligence and analytics maturity, according to Gartner.
Legacy systems often create more data silos than they resolve, making it essential to implement thoughtful governance and design strategies to modernize these systems and leverage orchestration tools effectively.
Section 2.2: The Importance of Data Orchestration
Data orchestration is critical for breaking down silos that inhibit data flow and freshness. While some companies may attempt to build DIY solutions, these often become obsolete as their stacks evolve, leading to wasted resources and frustration among engineers.
The advantages of data orchestration include:
- Enhanced Workflows for Engineers and Analysts: Utilizing data orchestration platforms automates data transformation and delivery, alleviating the burden on individual engineers and enabling real-time decision-making.
- Improved Data Governance and Visibility: Orchestration tools necessitate auditing and organizing the data stack, fostering greater visibility and confidence in data quality.
- Utilization of Fresh Customer Data: By automating processes, organizations can access up-to-date insights, enabling teams to better understand customer behavior and make informed decisions.
- Ensuring Data Privacy Compliance: With regulations like GDPR and CCPA, maintaining a clear documentation trail becomes challenging without a streamlined data stack. Data orchestration simplifies compliance efforts by providing comprehensive visibility into data collection processes.
In conclusion, data orchestration is an essential component for organizations looking to optimize their data management processes. By automating workflows and ensuring data accessibility, businesses can make informed, real-time decisions that drive success.
Thanks for Reading!
Be sure to SUBSCRIBE here to never miss another article on data science guides, tricks and tips, life lessons, and more!