The Challenge of Effective Algorithmic Auditing in A.I.
Written on
Understanding the Algorithmic Auditing Landscape
The discourse surrounding algorithmic auditing is gaining momentum as stakeholders recognize the need for a framework to evaluate the fairness of algorithms. This is particularly crucial as algorithms often disproportionately affect marginalized groups, including racial minorities, economically disadvantaged individuals, women, and people with disabilities, across various sectors such as healthcare, education, and employment.
Algorithms frequently function as statistical tools that analyze individual data to predict future outcomes—such as the likelihood of severe illness—leading to quantifiable “risk scores.” These scores guide decisions in resource allocation and service delivery, potentially influencing critical areas like healthcare and lending.
A promising development is the emergence of algorithmic auditing as a new field, with startups offering various forms of audits aimed at identifying bias and ensuring legal compliance in algorithmic models.
Section 1.1 The Role of Audits in Employment Algorithms
The need for algorithmic audits has become particularly pressing in the realm of automated hiring processes. For instance, New York City is currently debating Int. 1894–2020, a bill aimed at regulating automated employment decision-making tools, which mandates regular “bias audits” for such systems.
These tools—ranging from résumé parsers to social media analysis—are designed to help companies streamline their hiring processes, aiming to match the right candidate with the right position efficiently. The U.S. staffing and recruiting market was valued at approximately $151.8 billion in 2019, indicating the significant financial stakes involved.
As the economy recuperates from the Covid-19 pandemic, automated hiring tools will be pivotal in reshaping job access, particularly among communities disproportionately affected by unemployment, including Black, Latinx, and Asian residents.
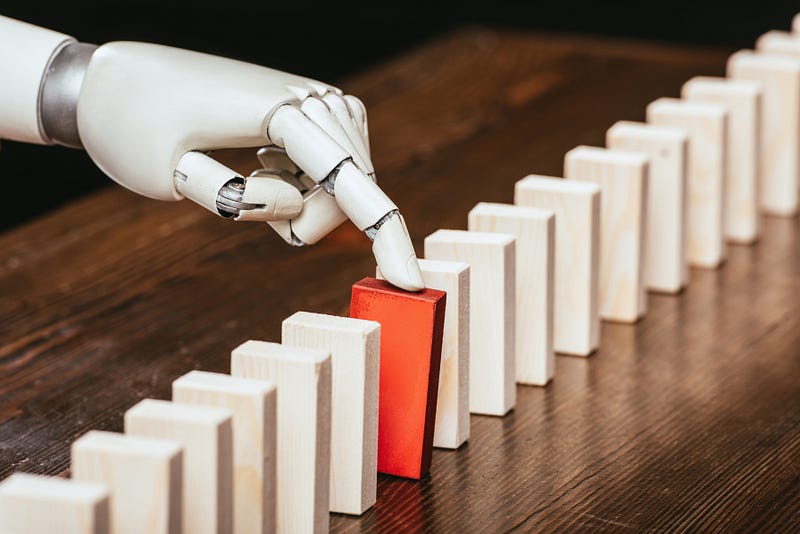
Section 1.2 The Pitfalls of Current Auditing Practices
Despite the positive intentions behind algorithmic audits, a significant challenge lies in the absence of a clear definition of what constitutes an “algorithmic audit.” While audits might sound rigorous, they can become mere tools for reputation management or, worse, legitimize harmful technologies based on unfounded pseudoscience.
Consider the case of physiognomy—an outdated belief that personality traits can be determined by physical appearance. In hiring contexts, this manifests in algorithms evaluating candidates based on facial expressions and other superficial metrics, raising serious ethical concerns.
In a recent incident, HireVue, a platform utilizing algorithmic assessments for hiring, reportedly misrepresented the findings of an audit conducted by O’Neil Risk Consulting. Despite claims of fairness, the audit’s effectiveness was compromised by restrictions imposed by HireVue on the auditors, highlighting the risks of biased auditing processes.
Chapter 2 Addressing the Flaws in Algorithmic Auditing
To tackle these issues, I propose three essential steps:
- Enhance Transparency: It is vital to disclose where and how algorithms are utilized in both public and private sectors. Applicants should be informed when automated tools are employed in hiring decisions. Cities like Helsinki and Amsterdam have initiated public registries for their algorithmic tools, which serve as models for transparency, although they face challenges due to vague definitions of key terms.
- Define Independent Audits: We must establish a comprehensive understanding of what constitutes an independent audit for automated decision-making systems. This includes identifying what aspects we audit and examining existing frameworks that have proven successful in various industries.
- Operationalize Algorithmic Auditing: It’s crucial to explore practical ways to implement algorithmic auditing effectively. Public procurement regulations, which account for 12% of global GDP, could integrate algorithmic auditability, fostering better practices and promoting the concept of “contestability by design,” allowing citizens to challenge outputs generated by these systems.
While the race to define effective algorithmic audits is ongoing, the goal is to ensure a fair and informed process that leads to equitable outcomes for all stakeholders involved.